In the world of e-commerce, personalized recommendations have become essential for enhancing user experience and boosting sales. By analyzing user behavior data, platforms can offer tailored shopping suggestions that improve satisfaction and conversion rates. Artificial intelligence (AI), particularly through machine learning and deep learning algorithms, has greatly advanced personalized recommendation systems. This article explores how businesses leverage AI for personalized recommendations, focusing on its principles, methods, advantages, and challenges.
I. The Principles of AI in Personalized Recommendations
The heart of a personalized recommendation system is understanding user needs and preferences. Traditional methods, like collaborative filtering and content-based filtering, have limitations, such as data sparsity and cold start issues. AI, especially through machine learning and deep learning, can more effectively capture and analyze user behavior patterns, leading to more accurate and personalized recommendations.
- Collaborative Filtering: This method can be user-based or item-based. User-based collaborative filtering recommends products based on the behaviors of similar users, while item-based does so based on the behaviors associated with similar items. AI enhances these algorithms, making them more effective at processing large amounts of data and improving accuracy.
- Content-Based Filtering: This approach recommends similar products based on a user’s past behavior and preferences. AI utilizes natural language processing (NLP) to analyze product descriptions, reviews, and other textual data, helping to identify product features and user interests.
- Hybrid Recommendation Systems: By combining collaborative filtering and content-based filtering, hybrid systems analyze both user behavior and product characteristics to enhance recommendation accuracy. AI can integrate these methods using deep learning models for better results.
II. Specific AI Methods for Personalized Recommendations
- Deep Learning Models: Deep learning uses multi-layer neural networks to learn complex features from large datasets. Common models include convolutional neural networks (CNNs) and recurrent neural networks (RNNs). For example, Amazon’s recommendation system employs deep learning to analyze user clicks, browsing, and purchase behaviors for tailored suggestions.
- Natural Language Processing (NLP): NLP techniques analyze product descriptions and user reviews. By employing sentiment analysis and topic modeling, platforms can gain deeper insights into user preferences and needs. For instance, analyzing user reviews can help identify specific demands for certain products, leading to more accurate recommendations.
- Reinforcement Learning: This method optimizes recommendation strategies through continuous experimentation and feedback.
- Graph Neural Networks (GNN): GNNs build a graph structure to analyze the relationships between users and products, effectively handling complex interactions and enhancing recommendation quality

III. Advantages of AI in Personalized Recommendations
- Enhanced User Experience: Personalized recommendations help users quickly find items they’re interested in, reducing search time and improving their shopping experience. Precise AI-driven suggestions can boost user satisfaction and loyalty.
- Increased Sales: By suggesting relevant products, AI can enhance conversion rates and average order values. For example, a recommendation system might suggest related accessories or similar items based on what’s in a user’s shopping cart, encouraging additional purchases.
- Improved User Retention: Continuous analysis of user behavior allows AI to refine recommendation strategies, enticing users to return and engage with the platform more often
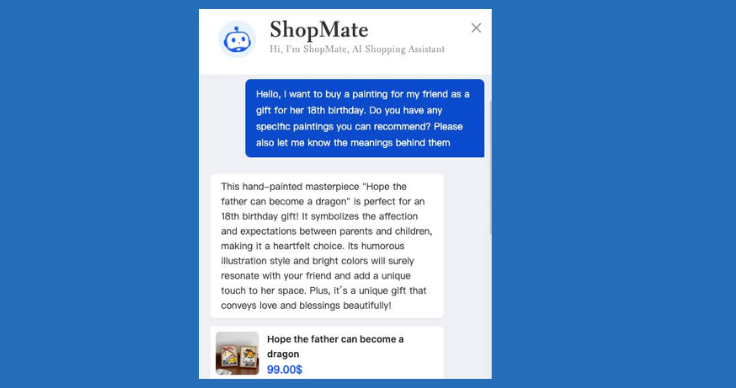
IV. Challenges Facing AI Technologies
- Data Privacy and Security: Personalized recommendation systems require significant user data, raising privacy concerns. E-commerce platforms must implement strong data protection measures to ensure user security and privacy, such as storing data securely.
- Data Sparsity and Cold Start Issues: New users or products often lack sufficient data for accurate recommendations. AI needs advanced algorithms to tackle these issues effectively.
- Algorithm Transparency and Interpretability: While deep learning models can achieve impressive results, they often lack transparency. E-commerce platforms need to ensure that their algorithms are understandable to users, fostering trust while improving recommendation accuracy.
- Technical and Resource Investment: Building and maintaining an effective AI recommendation system requires considerable technical expertise and resources. E-commerce platforms must find a balance between these investments and their business returns to ensure sustainability.
Conclusion
Artificial intelligence plays a critical role in personalized recommendations for e-commerce platforms. By leveraging technologies like deep learning, natural language processing, and reinforcement learning, AI significantly enhances recommendation accuracy and user experience. However, as the technology evolves, platforms must also address challenges such as data privacy and algorithm transparency. Looking ahead, AI is set to play an even more vital role in personalized recommendations, driving innovation and growth in the e-commerce industry.