In today’s fast-paced business world, supply chain operations are becoming increasingly complex. However, the integration of Artificial Intelligence (AI) and Machine Learning (ML) is revolutionizing the way these operations are managed. Let’s explore how these technologies are optimizing supply chain processes.
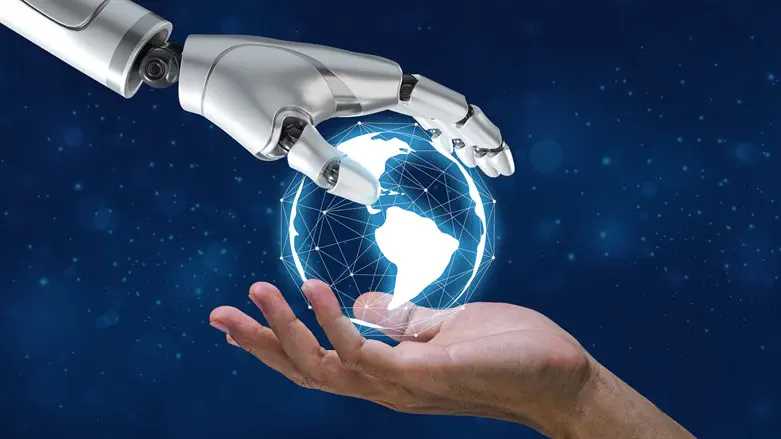
I. Demand Forecasting
A. Accurate Data Analysis
- Handling Big Data
AI and ML can analyze vast amounts of data from various sources. For example, they can gather data from historical sales records, customer behavior patterns, and even social media trends. By processing this big data, they can identify hidden patterns and trends that humans might miss. A clothing retailer can use these technologies to analyze past sales data, seasonal trends, and social media buzz about fashion to predict demand for different clothing items. - Real-time Data Processing
These technologies can process data in real-time. This means that supply chain managers can get up-to-date information about changes in demand. For instance, if there is a sudden increase in the popularity of a particular product due to a viral social media post, AI and ML can quickly detect this change and adjust the demand forecast accordingly.
B. Improved Forecasting Accuracy
- Reducing Forecast Errors
Traditional demand forecasting methods often have a high margin of error. But AI and ML algorithms can learn from past data and continuously improve their forecasting accuracy. For example, an electronics manufacturer can use ML algorithms to predict the demand for its new smartphone models. Over time, as the algorithms analyze more data, they can make more accurate predictions, reducing the risk of overstocking or understocking. - Accounting for Uncertainty
AI and ML can also account for various uncertainties in the market. They can consider factors such as economic changes, natural disasters, and political events that might affect demand. A food supply chain can use these technologies to anticipate disruptions in the supply of raw materials due to adverse weather conditions and adjust its demand forecast accordingly.
II. Inventory Management
A. Optimal Stock Levels
- Avoiding Overstocking and Understocking
AI and ML can help determine the optimal stock levels for products. By analyzing demand forecasts, lead times, and production capacities, they can suggest the right amount of inventory to keep. For example, a grocery store can use these technologies to ensure that it has enough stock of popular items like bread and milk, while also avoiding overstocking perishable goods. - Just-in-Time (JIT) Inventory
These technologies enable the implementation of Just-in-Time inventory strategies. They can predict when inventory levels will reach a critical point and trigger the replenishment process at the right time. An automotive manufacturer can use AI and ML to manage its inventory of parts, ensuring that the parts arrive just in time for production, reducing storage costs.
B. Inventory Visibility
- Real-time Tracking
AI and ML can provide real-time visibility into inventory levels. Using technologies like the Internet of Things (IoT), they can track the movement of goods throughout the supply chain. For example, a logistics company can use sensors on its trucks to monitor the inventory levels in transit and update the inventory records in real-time. - Predicting Inventory Shortages
They can also predict potential inventory shortages. By analyzing historical data and current trends, AI and ML algorithms can identify when a product is likely to run out of stock. A pharmaceutical company can use these technologies to predict shortages of essential drugs and take proactive measures to restock them.
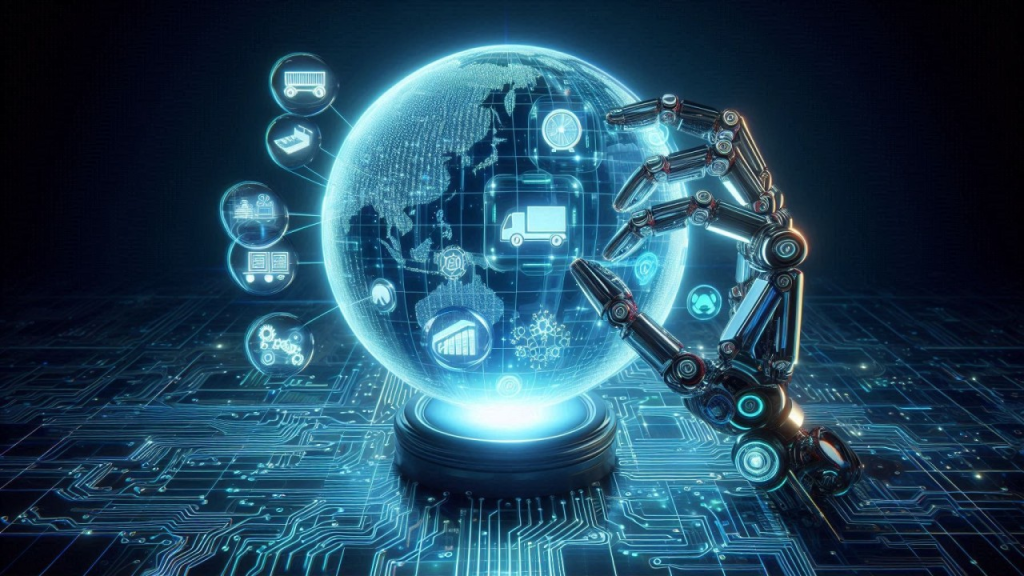
III. Logistics and Transportation
A. Route Optimization
- Finding the Best Routes
AI and ML can analyze multiple factors such as traffic conditions, delivery schedules, and vehicle capacities to find the best routes for transportation. For example, a delivery service can use these technologies to optimize the routes of its delivery trucks, reducing travel time and fuel costs. - Dynamic Route Adjustments
These technologies can also make dynamic route adjustments in real-time. If there is unexpected traffic or a road closure, AI and ML algorithms can quickly find an alternative route. A ride-sharing company can use these technologies to reroute its drivers to avoid traffic jams and ensure timely pickups and drop-offs.
B. Predictive Maintenance
- Preventing Equipment Failures
AI and ML can predict when transportation equipment such as trucks, ships, or airplanes are likely to experience failures. By analyzing data from sensors on the equipment, they can detect early signs of wear and tear. For example, an airline can use these technologies to predict when an engine component might fail and schedule maintenance in advance, preventing costly breakdowns during flights. - Reducing Downtime
By predicting maintenance needs, these technologies can reduce equipment downtime. A logistics company can use AI and ML to ensure that its delivery trucks are always in good working condition, minimizing disruptions to its supply chain operations.
IV. Supplier Management
A. Supplier Performance Evaluation
- Data-driven Assessment
AI and ML can evaluate supplier performance based on a wide range of data. They can analyze factors such as delivery times, product quality, and pricing. For example, a manufacturing company can use these technologies to assess the performance of its raw material suppliers and identify those that are consistently meeting its requirements. - Identifying High-risk Suppliers
These technologies can also identify high-risk suppliers. By analyzing historical data and market trends, AI and ML algorithms can predict which suppliers are likely to face issues such as financial problems or supply disruptions. A technology company can use these insights to diversify its supplier base and reduce the risk of supply chain disruptions.
B. Supplier Collaboration
- Enhancing Communication
AI and ML can improve communication between suppliers and buyers. They can use natural language processing to automate They can use natural language processing to automate communication processes and ensure that messages are clear and understood. For example, a procurement department can use these technologies to communicate with suppliers about order requirements and delivery schedules more effectively. - Joint Planning and Forecasting
These technologies can also facilitate joint planning and forecasting between suppliers and buyers. By sharing data and insights, they can work together to optimize the supply chain. A food manufacturer can collaborate with its ingredient suppliers using AI and ML to forecast demand and plan production more accurately.
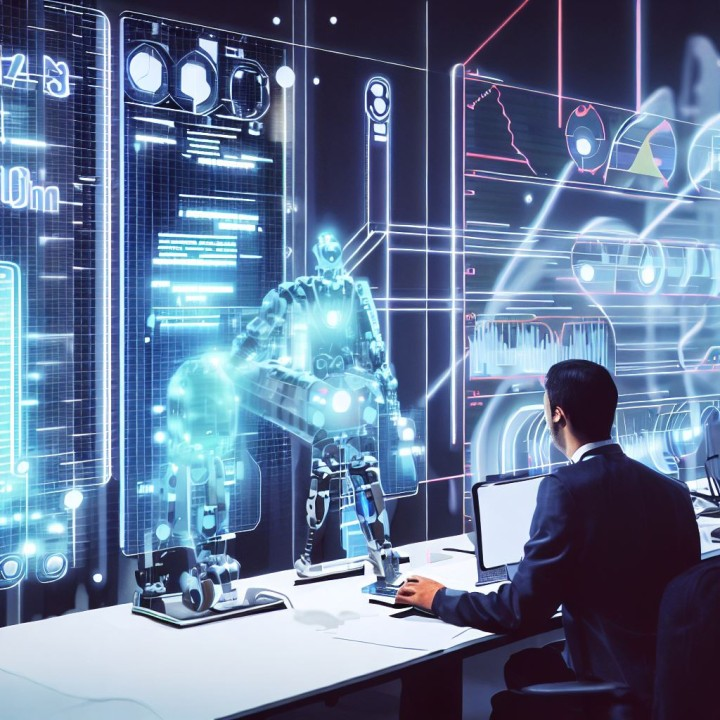
In conclusion, AI and Machine Learning are playing a crucial role in optimizing supply chain operations. From demand forecasting and inventory management to logistics and supplier management, these technologies are helping businesses improve efficiency, reduce costs, and enhance customer satisfaction. As technology continues to evolve, we can expect even more innovative applications of AI and ML in the supply chain industry.